1st prize of the year 2024
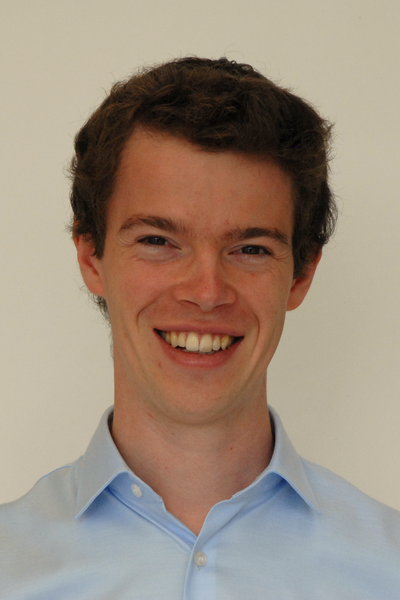
Interpretable deep learning for studying the Earth system / Soil-moisture–precipitation coupling across Europe
Dr Tobias Tesch
IBG-3: Agrosphere, Forschungszentrum Jülich
The Earth system consists of many variables that interact with each other on different time and space scales. For example, the relatively stable groundwater influences the chaotic precipitation. Due to the complexity of these interactions, many processes in the Earth system are not yet fully understood.
In his dissertation, Dr Tobias Tesch has developed an innovative approach to better understand the interaction of these variables: A deep learning model is trained to predict a target variable using other input variables. How the model makes these predictions is then analysed. While deep learning models can recognise complex relationships, these are often only statistical correlations and not causal relationships. Identifying and avoiding these spurious correlations is a major challenge.
Two new methods were developed in the current work: One that recognises spurious correlations and one that prevents the model from learning such spurious correlations. This is the first time that the "interpretable deep learning" approach has been combined with results from causality research.
These methods were used to investigate the influence of soil moisture on precipitation. A better understanding of this relationship helps to better predict and mitigate the effects of extreme weather events and the consequences of climate change. The new methods have provided important insights into this relationship, especially the importance of non-local effects, which have often been neglected in previous studies.
Dr Tobias Tesch's research therefore offers promising new approaches to better understand complex environmental processes and thus contribute to better weather and climate forecasts.